Clarifications
Clarification 1: Instruction includes fitting a linear function both informally and formally with the use of technology.Clarification 2: Problems include making a prediction or extrapolation, inside and outside the range of the data, based on the equation of the line of fit.
Benchmark Instructional Guide
Connecting Benchmarks/Horizontal Alignment
Terms from the K-12 Glossary
- Numerical Data
- Line of Fit
- Scatter Plot
Vertical Alignment
Previous Benchmarks
Next Benchmarks
Purpose and Instructional Strategies
In grade 8, students first worked with scatter plots and lines of fit. In Algebra I, students relate the slope and -intercept of a line of fit to association in bivariate numerical data and interpret these features in real-world contexts. In later courses, students use the correlation coefficient to measure how well a line fits the data in a scatter plot, and they also work with scatter plots that suggest quadratic and exponential models.- This is an extension of MA.912.DP.1.1, where students are working with numerical bivariate data (scatter plots and line graphs). It is good to review with students that a scatter plot is a display of numerical data sets between two variables.
- They are good for showing a relationship or association between two variables.
- They can reveal trends, shape of trend or strength of relationship trend.
- They are useful for highlighting outliers and understanding the distribution of data.
- One variable could be the progression of time, like in a line graph.
- In this benchmark, students are fitting a linear function to numerical bivariate data, interpreting the slope and -intercept based on the context and using that linear function to make predictions about values that correspond to parts of the graph that lie beyond or within the scatter plot.
- Instruction includes the use of technology for students to understand the difference between a line of fit and a line of best fit. Additionally, instruction of this benchmark should be combined with MA.912.DP.2.6 and MA.912.DP.2.5, as these are extensions of this benchmark.
- During instruction is important to distinguish the difference between a “line of fit” and the “line of best fit.”
- A “line of fit” is used when students are visually investigating numerical bivariate data that appears to have a linear relationship and can sketch a line (using a writing instrument and straightedge) that appears to “fit” the data. Using this “line of fit” students can estimate its slope and -intercept and use that information to interpret the context of the data.
- The “line of best fit” (also referred to as a “trend line”) is used when the data is further analyzed using linear regression calculations (the process of minimizing the squared distances from the individual data values to the line), often done with the assistance of technology.
Common Misconceptions or Errors
- Students may not know how to sketch a line of fit.
- For example, they may always go through the first and last points of data.
- Students may be confuse the two variables when interpreting the data as related to the context.
- Students may not know the difference between interpolation (predictions within a data set) and extrapolation (predictions beyond a data set).
Strategies to Support Tiered Instruction
- Teacher provides sketched lines of fit and has students identify the one that best models the data.
- Teacher provides a sentence frame for interpreting the data in the context of the problem using two different colored highlighters to highlight the same variable in the sentence frame and table or graph.
- Example:
- Example:
- Teacher models creating a scatter plot on a piece of graph paper, then has students place a piece of spaghetti on the scatter plot to model the line of best fit. Students could also use a coordinate plane peg board to plot each point creating a scatter plot and then use a rubber band to model the line of best fit.
- Instruction includes vocabulary development by co-creating a graphic organizer for interpolation and extrapolation.
Instructional Tasks
Instructional Task 1 (MTR.4.1, MTR.5.1, MTR.6.1)- Crickets are one of nature’s more interesting insects, partly because of their musical ability. In England, the chirping or singing of a cricket was once considered to be a sign of good luck. Crickets will not chirp if the temperature is below 40 degrees Fahrenheit (°F) or above 100 degrees Fahrenheit (°F). A table is given with some data collected.
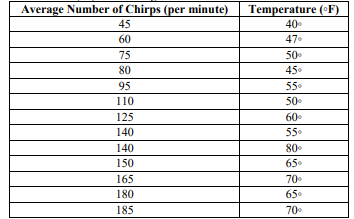
- Part A. Create a line of fit based on the data. Compare your line of fit with a partner.
- Part B. What is the estimated slope and -intercept of the line?
- Part C. What does the slope mean in terms of the context?
- Part D. What does the -intercept mean in terms of the context?
- Part E. Using technology, determine the line of best fit. Compare this to the line of fit determine from Part A. What is the difference?
- Part F. Based on this line, predict the temperature to be if you recorded 250 chirps per minute?
- Part G. Based on this line, estimate the number of chirps per minute at exactly 50°F.
Instructional Items
Instructional Item 1- Below is data from a variety of fast food chains.
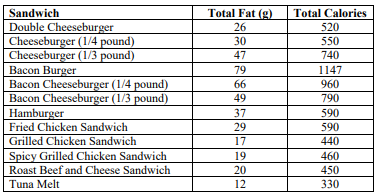
- Part A. Create a scatter plot based on the data above and estimate an equation for a line of fit.
- Part B. What do the slope and -intercept tell us about the relationship of total fat and total calories in these fast food items?
- Part C. If a fast food item has 10 grams of fat, estimate the total calories of that item.
Related Courses
Related Access Points
Related Resources
Formative Assessments
Lesson Plans
Perspectives Video: Experts
Perspectives Video: Professional/Enthusiasts
Problem-Solving Task
STEM Lessons - Model Eliciting Activity
Students will apply skills (making a scatter plot, finding Line of Best Fit, finding an equation and predicting the y-value of a point on the line given its x-coordinate) to a fuel efficiency problem and then consider other factors such as color, style, and horsepower when designing a new coupe vehicle.
Model Eliciting Activities, MEAs, are open-ended, interdisciplinary problem-solving activities that are meant to reveal students’ thinking about the concepts embedded in realistic situations. Click here to learn more about MEAs and how they can transform your classroom.
MFAS Formative Assessments
Students are asked to interpret the meaning of the constant term in a linear model.
Students are asked to interpret the line of best fit, slope, and y-intercept of a linear model.
Students are asked to informally fit a line to model the relationship between two quantitative variables in a scatterplot, write the equation of the line, and use it to make a prediction.
Students are asked to interpret the intercept of a linear model of life expectancy data.
Students are asked to interpret the meaning of the slope of the graph of a linear model.
Students are asked to interpret the meaning of the slope of the graph of a linear model.
Students are asked to use a linear model to make and interpret predictions in the context of the data.
Students are asked to use a linear model to make a prediction about the value of one of the variables.
Student Resources
Problem-Solving Task
The purpose of this task is to assess ability to interpret the slope and intercept of the line of fit in context.
Type: Problem-Solving Task
Parent Resources
Problem-Solving Task
The purpose of this task is to assess ability to interpret the slope and intercept of the line of fit in context.
Type: Problem-Solving Task